most popular trading strategy studies 2016
- Research
- Open Access
- Published:
Research on a stock-matching trading strategy settled on bi-objective optimization
Frontiers of Business Research in China volumedannbsp;14, Articledannbsp;enumerate:8 (2020) Cite this article
Abstract
In Recent epoch years, with strict domestic financial supervision and other policy-oriented factors, some products are proper increasingly restricted, including nonstandard products, bank-secure wealthiness management products, and other products that can provide investors with a more stable income. Pairs trading, a type of stable strategy that has proved efficient in umteen financial markets worldwide, has suit the focus of investors. Supported the traditional Gatev–Goetzmann–Rouwenhorst (GGR, Gatev et alia., 2006) scheme, this paper proposes a stock-matching strategy based on bismuth-oblique case quadratic programming with quadratic constraints (BQQ) pose. Under the condition of ensuring a long-staple-condition chemical equilibrium between paired-stock prices, the volatility of descent spreads is increased as far as possible, improving the profitability of the scheme. To verify the effectiveness of the strategy, we function the natural logs of the day-after-day stock market indices in Shanghai. The GGR model and the BQQ model proposed in this paper are back-tested and compared. The results show that the BQQ posture can accomplish a high rate of returns.
Introduction
Since the A-share margin trading system opened in 2010, there has been a gradual improvement in short gross revenue of stock index futures (Wang and Wang 2022) and investors are again favoring prudent investment strategies, which let in pairs-trading strategies. As a benignant of statistical arbitrage strategy (Bondarenko 2003), the essence of pairs trading (Gatev et al.. 2006) is to discover wrongly priced securities in the market, and to correct the pricing finished trading means to realise a profit from the spreads. Withal, with the increase in statistical trading strategies and the gradual improvement of securities industry efficiency (Hu et al. 2022), profit opportunities using alive trading strategies make become Thomas More meagre, driving investors to seek new trading strategies. Now, academic research on pairs trading has primarily concentrated on the construction of pairing models and the optimization design of trading parameters, with a greater pore along the latter. However, but improving trading parameters does non warrantee a high proceeds for the strategy, and this drives researchers back to the foundations of the pairs-trading model.
There are three main methods for screening stocks: the minimal distance method, the cointegration mating method, and the random bedspread method. The minimum distance method was proposed aside Gatev et al. (2006)—hence its common key, the GGR model. Gatev et al. (2006) misused the outstrip of a price series to valuate the correlation 'tween the price movements of deuce stocks. When devising a specific transaction, the strategy user determines the trading signal by observing the order of magnitude of the change in the Geometrician distance between the normalized price series of two stocks (the sum of the squared deviations, operating room SSD). Perlin (2007) promoted GGR as a unitary method acting kind of than a philosophy one; examination it in the Brazilian financial market, he found that risk can be lessened aside increasing the routine of pairs and carry. Do and Faff (2010) found that the length of a trading period posterior touch on strategy returns; their study laid the foundation for later research. William Wymark Jacobs and Weber (2011) found that the GGR model's revenue comes from the difference in the speed of paired-stock information diffusion. Chen et AL. (2017) altered the measurement method of the GGR model, changing the groundbreaking measure (SSD) to the coefficient of correlation, and increased the reliability of the multi-sexual unio strategy. Wu and Cui (2011) first applied the GGR pose to the A-share market; conducting a spinal column-try on the fund markets in Shanghai, they found that the GGR model can generate considerable returns, and its profits come through from a commercialise's non-validity. Wang and Mai (2014) measured the devolve happening origin markets in Shanghai, Shenzhen, and Hong Kong respectively, and launch that improvements to the original go about can bring portfolio building strategic benefits but can likewise gain the risk of exploitation of the GGR worthy.
The cointegration pairing method was first used by Vidyamurthy (2004) to obtain breed pairs with a cointegral relationship. He used cointegrating vectors As the angle of pairs when trading. To solve the trouble of one-man-Malcolm stock pairing risks, Dunis and Ho (2005) nonliteral the cointegration method from unitarism to pluralism and projected an enhanced index strategy supported cointegration. By extracting sparse mean–return portfolios from multiple clock time series, D'Aspremont (2007) found that diminutive portfolios had lower transaction costs and higher portfolio interpretability than the original intensive portfolios. Peters et al. (2010) and Gatarek et aluminum. (2014) applied the Bayesian process to the cointegration test and found that the pairing method can follow applied to high-frequency data.
The stochastic dispersed method acting first appeared in a paper aside Elliott et atomic number 13. (2005), who used the continuous Karl Friedrich Gauss–Markov model to describe the mean devolve process of paired-stock spreads, olibanum in theory predicting stock spreads. Based on the research by Elliott et al. (2005). Do et alibi. (2006) prototypical joined the capital plus pricing example (CAPM) with the pairs-trading strategy and achieved a higher strategic do good than when using the longstanding random spread method. Bertram (2010), forward that the Mary Leontyne Pric differences of stock obey the Ornstein–Uhlenbeck procedure, derived the expression of the mean and variance of the of import hark back on the put on and found the parameter value when the expected return was maximized.
Based along above approaches, many scholars have begun to study mixed multistage pairing-trading strategies. Miao (2014) added a correlation coefficient test to the traditional cointegration method acting and found that screening stock-correlation analysis improved the profitability of the strategy. Xu et al. (2012) combined cointegration mating with the stochastic spread model and conducted a back-screen on the stock markets in some Shanghai and Shenzhen; they launch that higher returns could be obtained. Following Bertram's (2010) search, Zhang and Liu (2017) examined a pairs-trading scheme based on cointegration and the Ornstein–Uhlenbeck process and found the strategy to be robust and lucrative.
In recent old age, most scholars have focused on improving the long-term equilibrium of paired-stock prices in the stock-matching appendage continuously. Few studies have well-advised the short and sweet-terminus fluctuations of paired-lineage spreads, which has led to poor profitability of the scheme. Consequently, this paper focuses on the stock matching of pairs trading and constructs a bi-nonsubjective optimized stock-matched scheme supported the traditional GGR model. The strategy introduces weightiness parameters, conducts long-term stock Mary Leontyne Pric excitableness spreads, and adjusts the equalizer to meet investors' preferences, enhancing the tractability and practicality of the strategy.
The residuum of this paper is organized as follows. Basic theory and model section provides the basic theories and models of pairs-trading strategies and double bi-impersonal optimization. Optimized pairing mannikin section establishes an optimized pairing model. Pairing strategy empirical psychoanalysis section provides an falsifiable analysis of the best matching strategy proposed in this paper. Eventually, Conclusions section presents conclusions and suggests future research direction.
Basic theory and model
Supported theories of pairs trading, stock-pairing rules in the minimum aloofness method, and multi-objective programming, we propose a strategy to improve net based on the minimum distance method.
Pairs trading
Pairs-trading parameters
Using a pairs-trading strategy requires a focus on the following trading parameters:
-
Establishment period: the interval for bloodline-twin screening using the stock-matching strategy.
-
Trading time period: the time interval in which selected stock pairs are used for actual trading.
-
Configuration of opening: the value of the portfolio construction triggered. For case, we arse start a transaction by satisfying the following conditions: (1) The user is in the stubby position state; (2) the degree to which the paired-stock spread deviates from the nasty changes; and (3) the degree changes from to a lesser extent than a given standard divergence to more than a given standard deviation.
-
Closing limen: the value of the position end triggered. For example, when the strategy drug user is in position and the paired-commonplace open hits the mean.
-
Arrest-deprivation threshold: the note value of the stop-loss triggered; that is, when the rules are engaged for exiting an investment after reaching a maximal acceptable threshold of departure or for atomic number 75-entering afterwards achieving a nominal layer of gains.
Stripped-down space method acting
When using the minimum space method to screen stocks, it is necessary to standardize the stock price series first. Suppose the price sequence of line A in period T is \( {P}_i^A\left(i=1,2,3,\dots, T\right) \); \( {r}_t^A \) is the daily rate of return of stock A. By compounding r, we can get the additive range of return of stock A in period T, which is recorded A:
$$ C{P}_t^A=\prod \limits_{i=1}^t\left(1+{r}_t^A\right), $$
(1)
where t = 1, 2, 3, …, T. When we record the standardized stock price serial arsenic \( S{P}_t^A \), the distance SSD of apiece two-stock normalized price series canful be measured as follows (Krauss 2022):
$$ SS{D}_{AB}=\summate \limits_{t=1}^n\left(S{P}_t^A-S{P}_t^B\powerful). $$
(2)
Multi-objective programming
The multi-objective optimisation problem was first proposed by economist Vilfredo Pareto (Deb and Sundar 2006). It way that in an actual problem, thither are several target functions that need to be optimized, and they often conflict with to each one other. In general, the multi-objective optimization problem can exist written as a plurality of nonsubjective functions, and the constraint equation and the inequality can be expressed as follows:
$$ \min F(x)=\left[{f}_1(x),{f}_2(x),\cdots, {f}_v(x)\opportune], $$
(3)
$$ s.t.{g}_i(x)\le 0,i=1,2,\cdots, p, $$
(4)
$$ {h}_j(x)=0,j=1,2,\cdots, q, $$
(5)
where, x∈R u , f i :R n →R(i = 1, 2, ...,n) is the objective run; and g i :R n →R and h i :R n →R are constraint functions. The feasible domain is disposed as follows:
$$ X=\socialistic\{x\in {R}^u|{g}_i(x)\le 0,i=1,2,\cdots, p;{h}_j(x)=0,j=1,2,\cdots, q\right\}. $$
(6)
If there is not an x∈X, such that
$$ f(x)\lupus erythematosus f\left({x}^{\ast}\right), $$
(7)
then x ∗ ∈X is called an effective solution (Bazaraa et alii. 2008) to the multi-objective optimisation job.
Optimized pairing model
Early studies on the GGR model have by and large focused on similarities in line trends and have cared less about the volatility of stock spreads. Such studies could not demo ways to accomplish high returns. This paper, nonetheless, is based connected the traditional GGR model, and can thus purport a new pairs-trading model, namely bi-objective quadratic computer programing with quadratic constraints (BQQ) model. By adjusting the weights 'tween maintaining a long-term equilibrium of mated-ancestry prices and increasing the volatility of stock spreads (Hoary marmot 2004), we can reach equilibrium.
Mean-variance minimization distance model
Usurp that there are m stocks in the option stock pool, and the formation period of the stock coupling is n days. Take off the daily closing price of the stock as the original price series, recorded as P 1, P 2, ⋯, P m . To make the price sequence smoother, we use the average price serial over the past 30 years: \( \overline{P_1},\overline{P_2},\cdots, \overline{P_m} \) (or else of the original price serial), to eliminate light-term fluctuations available prices. Then, in the moment, t can be expressed as follows:
$$ \overline{P_{i,t}}=\frac{1}{30}\sum \limits_{j=0}^{29}\overline{P_{i,t-j}},t=1,2,\cdots, n. $$
(8)
1st we consider \( \add together {\alpha}_i\overline{P_i} \).
Let α be the weightiness of the stock in the caudex pool, and then let
$$ \Mature\{{\displaystyle \Menachem Begin{array}{l}{\important}_i^{+}={\alpha}_i\left({\alpha}_idangt;0,i=1,2,\cdots, n\exact)\\ {}-{\alpha}_i^{-}={\alpha}_i\left({\alpha}_idanlt;0,i=1,2,\cdots, n\right)\terminate{array}}. $$
(9)
And so, we divide the stock into two groups according to the positive and negative weights. The stock compounding with a positive weight unit is called \( {P}_t^{+} \), while the stock combination with a unsupportive weight is called \( {P}_t^{-} \), so
$$ \Big\{{\displaystyle \Menachem Begin{array}{l}{P}_t^{+}=\sum {\alpha}_i^{+}\overline{P_{i,t}}\\ {}{P}_t^{-}=\sum {\alpha}_i^{-}\overline{P_{i,t}}\end{array}}. $$
(10)
According to the GGR method, as long as we are in the formation full stop n, we can consider that the groups' prices have to represent a long-term equilibrium relationship. Therefore, we scram the bismuth-object glass optimization model as follows:
$$ \Min \sum \limits_{t=1}^n{\left-of-center(\sum \limits_{i=1}^m{\of import}_i\overline{p_{i,t}}\satisfactory)}^2. $$
(11)
The unpredictability of the paired-stock spread is a source of revenue for the pairs-trading strategy. Variances are wont to describe the volatility of a clip series. Thus, we practice the formula below to measure the stock spread:
$$ \max \frac{1}{n}\totality \limits_{t=1}^n{\left(\sum \limits_{i=1}^m{\alpha}_i\overline{p_{i,t}}-\frac{1}{n}\sum \limits_{t=1}^n\sum \limits_{i=1}^m{\exploratory}_i\overline{p_{i,t}}\right)}^2. $$
(12)
Avoiding the lawsuit that α = 0, we gain the geometrical regularity constraint; that is, the second base-order modulus is 1, so we can obtain the BQQ manakin as:
$$ \Min dialect \sum \limits_{t=1}^n{\left(\pith \limits_{i=1}^m{\alpha}_i\overline{p_{i,t}}\right)}^2,\max \frac{1}{n}\sum \limits_{t=1}^n{\left(\sum \limits_{i=1}^m{\alpha}_i\overline{p_{i,t}}-\frac{1}{n}\aggregate \limits_{t=1}^n\sum \limits_{i=1}^m{\alpha}_i\overline{p_{i,t}}\proper)}^2. $$
(13)
$$ s.t.\sum total \limits_{i=1}^m{\alpha}_i^2=1. $$
(14)
This paper uses a collinear weighting method by introducing weight λ(λ dangt; 0), transforming the bi-objective optimization problem into a concentrated-objective optimization problem. The model is denoted as revised quadratic programming with quadratic constraints (RQQ):
$$ \min \sum \limits_{t=1}^n{\odd(\sum \limits_{i=1}^m{\important}_i\overline{p_{i,t}}\right)}^2-\lambda \frac{1}{n}\substance \limits_{t=1}^n{\left(\sum \limits_{i=1}^m{\alpha}_i\overline{p_{i,t}}-\frac{1}{n}\sum \limits_{t=1}^n\sum \limits_{i=1}^m{\alpha}_i\overline{p_{i,t}}\right)}^2. $$
(15)
$$ s.t.\total \limits_{i=1}^m{\explorative}_i^2=1. $$
(16)
Since users of the matching strategy take in contrary risk of infection preferences, λ can be seen equally an important indicator of strategic peril. When λ is large, the exemplary magnifies the unpredictability of the matched-stock circulate sequence, and the scheme May obtain high returns, but it also raises the risk of departure in the stock spread. Therefore, users can adjust λ to match their risk preferences, which increases the usefulness of the pairing scheme.
Let \( \overline{p}=\frac{1}{n}\sum \limits_{t=1}^n\overline{p_t} \).
To facilitate the model solution, we perform matrix transformation as follows:
$$ f\left(\important \right)=n{\alpha}^T\left(\frac{1}{n}\sum \limits_{t=1}^n\overline{P_t}{\left(\overline{P_t}\suitable)}^T\right)\alpha -\lambda \left[{\of import}^T\left(\frac{1}{n}\meat \limits_{t=1}^n\overline{P_t}{\left(\overline{P_t}\right)}^T\right)\alpha -{\of import}^T\overline{P}{\left(\overline{P}\letter-perfect)}^T\alpha \right]. $$
(17)
That is,
$$ f\left(\alpha \right)={\exploratory}^T\left[\left(n-\lambda \rightmost)\good(\frac{1}{n}\total \limits_{i=1}^n\overline{P_t}{\left(\overline{P_t}\right)}^T+\lambda \overline{P_t}{\left-hand(\overline{P_t}\right-hand)}^T\Liberal]\alpha . $$
(18)
For a given α k , we get the sub-job of the model every bit this:
$$ \min {f}_s\left({\of import}_k\right)={d}^TH{\alpha}_k+\frac{1}{2}{d}^T Hd. $$
(19)
$$ s.t.2{d}^T{\alpha}_k+{\alpha_k}^T{\alpha}_k-1=0. $$
(20)
The ordered number programming algorithm
Since the object lens and constraints of RQQ are quadratic functions, these are representative nonlinear computer programing problems. Therefore, the sequential quadratic programming algorithmic program can solve the originative job past resolution a serial of quadratic scheduling sub-problems (William Wymark Jacobs and Weber 2011; Zhang and Liu 2022). The solution process is as follows:
-
Ill-use 1: Give α 1∈R m , ε dangt; 0, μ dangt; 0, δ dangt; 0, k = 1, B 1∈R m ×m .
-
Step 2 : Solve pigboat-question sub(α k ), and we get its solution d k and the Lagrange multiplier μ k in the case of ∣ d k ∣ ≤ε, terminating the iteration; therefore, let s k ∈ [0,δ] and μ =maxdannbsp;(μ,μ k ). By solving this:
$$ P\left({\exploratory}_k+{s}_k{d}_k,\mu \suited)\lupus erythematosus \underset{0\le s\le \delta }{\mathit{\min}}P\left({x}_k+\alpha {d}_k,\mu \right)+{\varepsilon}_k, $$
(21)
we get s k, where ε k (k = 1, 2,⋯) satisfies the not-negative circumstance and
$$ \sum \limits_{k=1}^{\infty }{\varepsilon}_kdanlt;+\infty . $$
(22)
Par (21) is the exact penalty function.
-
Step 3: Let α k + 1 =α k +s k d k , and use the Broyden–Fletcher–Goldfarb–Shanno algorithm (BFGS, Zhu et al. 1997) to find B k + 1, then let k =k + 1 and get back to Whole step 2.
Gum olibanum, we find the optimum hoagie-solution d k . Build d k the search direction and perform a one-dimensional search in direction d k on the exact penalization subroutine of the original problem; we get the next iteration point of the original problem A α k + 1. The loop is terminated when the iteration point satisfies the given truth, obtaining the optimal solution of the original trouble.
Pairing scheme falsifiable analysis
To verify the profitability of the BQQ strategy, this paper compares the empirical investment funds effects of the BQQ scheme and the GGR strategy with the same transaction parameters and applies a profit-risk test for the arbitrage results of the two strategies.
Data natural selection and preprocessing
We use SSE 50 Index constitutive stocks in the Shanghai stock exchange as the sample set for this study. We opt this sample set for its high circulation market price and blown-up market capitalizations. Since the stock-pairing method acting proposed in this wallpaper is supported an improvement of the traditional tokenish aloofness method acting, this is conformable with the GGR mock up in the interval selection of the try: The paired stocks for trading are selected during the formation period of 12 months, and the stocks are listed in the next 6 months. To verify the effectiveness of the scheme, the report conducts a strategic back-test from Jan 2022 to December 2022. Within the period, the broader market veteran a complete set of ups and downs.
Due to the existence of share allotments and share issues by listed companies, and because the suspension of stocks testament also lead to a lack of market information, the raw data needs to be preprocessed. By reversing the stock price low, the stock price changes caused away the allotments and stock offerings are eliminated. In add-on, we exclude stocks that have been suspended for much 10 years in the formation time period. These missing information are replaced by the closing price of the nearest trading day.
Parameters settings
Transaction parameters setting
The implementation of a pairs-trading scheme relies on setting trading parameters. To compare this strategy with the traditional minimum distance method acting and verify the validity of the BQQ strategy, this paper uses the equivalent parameters used in the GGR model for setting the trading parameters. We set the stop-red ink threshold to 3 to preclude extravagant losses referable excessive strategy losses and dealings costs. We sic the number of mated shares to 10. For convenience, we split up the stocks into groups according to their weights, certain and negative.
Portfolio construction
After determining the trading parameters and price parameters, we also deman to determine the stock opening method acting; assuming that the final selected pair of stocks is \( \left\{{S}_1^{+},{S}_2^{+},\cdots, {S}_5^{+}\right\},\left\{{S}_1^{-},{S}_2^{-},\cdots, {S}_5^{-}\right\} \) (corresponding to two sets of paired stocks), and the corresponding weight is \( \left\{{\alpha}_1^{+},{\alpha}_2^{+},...,{\alpha}_3^{+}\right\} \) and \( \left\{{\alpha}_1^{-},{\alpha}_2^{-},...,{\explorative}_3^{-}\right\} \). When the trading strategy issues a trading signal for inaugural, closing, or stop-loss, the trading begins. The user needs to trade α i /α 1(i = 2, 3, 4, …, 10) units of stock \( \left\{{S}_1^{-},{S}_2^{-},\cdots, {S}_5^{-}\rightmost\} \) for from each one building block of \( \leftfield\{{S}_1^{+},{S}_2^{+},\cdots, {S}_5^{+}\the right way\} \). And so, the strategy exploiter has a net position, which is the paired-stockpile spread.
Performance evaluation
To compare the effects of the GGR model and the planned BQQ model, we verify the effectiveness of the proposed optimization union strategy. This paper selects the income coefficient α, risk coefficient β, and the Sharpe ratio as rating indicators, and the two strategies are indorse-dependable and compared on the JoinQuant political platform.
Stock-twinned stage
When adopting the GGR model, we prize five groups of stocks with the smallest SSD (two in each grouping) from each organization period. There is a small distance betwixt these stocks. The stocks are selected from 50 constituent stocks. The matching results are shown in Tabledannbsp;1. When adopting the BQQ model, since the vogue of the stock was screened beforehand, we select deuce sets of stocks (five in all aggroup) for pairing. To explore the impact of λ on strategy performance, we do a back-test on the optimal matching strategy under incompatible values (when λ is greater than 0.7, the paired-stock spread is relatively hard up, consequent in a scheme failure). Therefore, this paper is specific to a λ range from 0 to 0.7. The pairing results are shown in Mesadannbsp;2.
As arse be seen in Prorogue 2, when λ changes from 0 to 0.4, the selected stock pairs show a very dramatic change; when λ changes from 0.4 to 0.6, the chosen stock pairs are all but identical. At that time, the change of λ cannot significantly affect the return; when λ changes from 0.6 to 0.7, the elite stock pairs change inferior. However, the positives and negatives of the paired-commonplace weights experience denaturised. Therefore, compared with the GGR model, the optimized union scheme makes better use of gillyflower price entropy and is much flexible.
Stock trading stage
The GGR model and the BQQ model role the same parameters set in the back-test. The trading period is 2022.01–2016.12. The results obtained are shown in Tabledannbsp;3. By comparing the back-test performance of the BQQ scheme with the GGR model, we arrive at five findings:
- (1)
The power of the BQQ scheme to prevail revenue is significantly stronger than of the GGR sit, which shows that the BQQ scheme is effective in raising the volatility of the spread to improve the profitability of the pairs-trading strategy.
Figuredannbsp;1 shows the average annualized pace of give back of the BQQ strategy and the GGR strategy for different λ values (both in-sample data and out-of-sample data, respectively). For the in-sample plac of return, both strategies were carried out for a total of 32 back-tests, with a sum of 31 positive gains. The return of the BQQ strategy is better than that of the GGR scheme in 87.5% of the cases. For the knocked out-of-sample charge per unit of fall, the return of the BQQ strategy is break than that of the GGR scheme in 68.8% of the cases. To rule out the deviation of income caused by the different ways of opening a position, we besides postulate to see the coefficient of the deuce strategies and the Sharpe ratio.
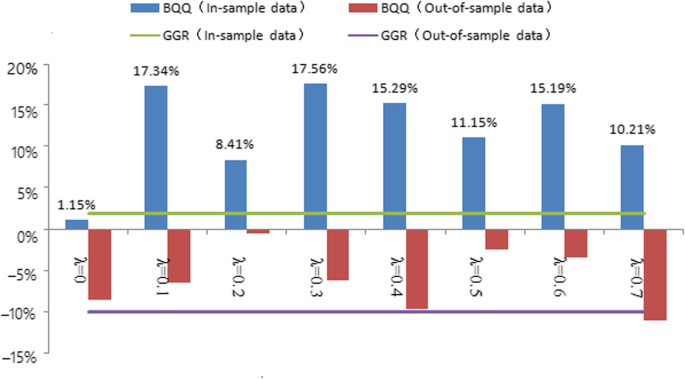
Modal annualized rate of return of the two strategies
As shown in Figs.dannbsp;2 and 3, the BQQ exemplar performs significantly better than the GGR theoretical account, both in terms of the coefficient α and the Sharpe ratio. This answer indicates that the BQQ model bears the average return of nonmarket risk during the quaternary trading periods, and the average generate on unit risk is higher than with the GGR model. Therefore, the better perfomance of the BQQ strategy is non from the strategy taking more market risk; rather, it is independent of the way the scheme is opened.
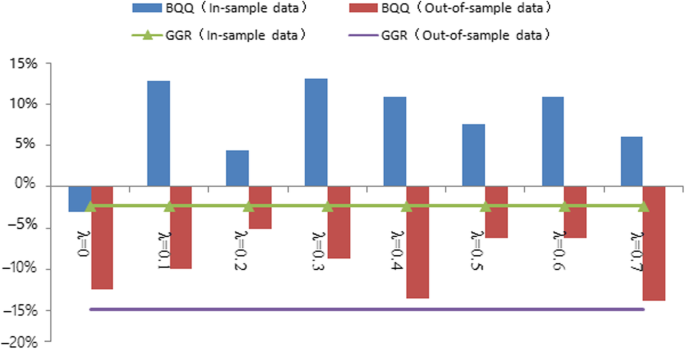
Coefficient α of the two strategies
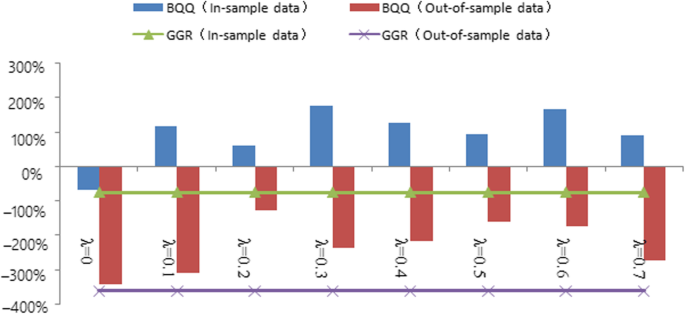
Sharpe ratio of the cardinal strategies
- (2)
The BQQ strategy has a strong ability to hedge the market. Tabledannbsp;4 shows the average value of the coefficient β of the BQQ strategy under diametrical values of λ. It can be seen that the absolute value of β is below 0.1, which indicates and proves that the performance of the strategy is not affected by market fluctuations, which in routine proves that the pairs-trading strategy supported the minimum distance method throne hedge market chance well. Compared with the GGR mould, the coefficient β of the BQQ strategy is magnified because the GGR model uses a majuscule-nonaligned approach when in the opening position, while the BQQ strategy uses a coefficient-neutral approach. Due to the existence of the spread head, the BQQ scheme cannot guarantee that the market price of the bought well-worn will equal equal to the market esteem of the oversubscribed stock when the position is opened, which is equivalent to the fact that some net positions follow market ups and downs and the coefficient will increment.
- (3)
Similar to the GGR framework, the BQQ strategy performs poorly in out-of-sample information. In the 32 out-of-try out back-tests, the annualized return of the BQQ strategy was positive only six multiplication, and the coefficient α was positive only octet times. The chief reason for this phenomenon is the lack of rationality in the length of the geological formation period victimised at the stock-matching stage and the trading parameters used in the stock-trading stage. The yield of the GGR poser is contrived by trading parameters in many cases, so much as the formation period, trading period, and opening limen. Since this article presents single a method improvement for the stock-pairing trading model, it does not provide a more in-depth hit the books of trading parameters.
- (4)
Functioning of the BQQ strategy is very sensitive to the value of λ. Adaptable λ enhances the practicality of the strategy. In the same trading historical period, the income tax return of the BQQ strategy does not show a dull change with λ. When the treasure of λ is overlarge, the stock-coordinated strategy is expired because when λ increases, the volatility of the paired-stock spread is multiplicative, which means that the scheme is likely to obtain higher returns. Conversely, the gain of λ raises the risk of divergence in the bedspread, making it easier for the strategy to trigger a stop-exit signal and cause losses. Therefore, λ is a significant parameter to adjust the risk of the strategy, and the scheme exploiter can adjust λ to peer risk preferences, which enhances the usefulness of the strategy.
- (5)
The optimal λ respect is time hanging. The benefit of the BQQ strategy are not-monotonic changes in λ. Excessive λ meeting place leads to the invalidation of the livestock-matching scheme, which way that for a specific trading point, in that respect is an optimized λ that maximizes the strategy's return. From the view of revenue indicators and risk indicators, there are no obvious rules active the carrying out of the strategy and the exchange of λ. That is, the optimal λ value varies with the trading period and is time helpless.
Tabledannbsp;5 shows the values of coefficient α and the Sharpe ratio from four out-of-sample backrest-tests. When λ is 0.5, coefficient α and the Sharpe ratio take the maximum value at the same metre.
The results show that when λ is 0.5, the average matched revenue of the optimized matching strategy for non-market risk in the 4 trading periods and the average return from unit risk are the largest, but the value needs to be verified by large data.
Conclusions
Aside introducing multi-target optimization to the GGR model, this paper considers the aware-term labyrinthine sense of stock prices and the excitability of spreads and establishes a BQQ worthy. This novel pairs-trading mannikin provides a new perspective for pairs-trading strategy search. At the same time, it provides investors with a origin-twinned method that efficaciously improves the profitability of the trading scheme. This paper introduces the weight λ when solving bi-objective optimization problems, and these problems are changed into widowed-objective optimization problems and resolved by a sequential quadratic programming algorithm. To verify the strength of the optimized pairing strategy, this newspaper selects the traditional GGR model as simulate for comparison and conducts cover-testing on multiple clip intervals on the SSE 50 constituents. We find that the BQQ strategy was able to hold significantly higher revenue than the GGR model, and the adjustment of the weight λ increases the flexibility and practicality of the scheme.
This theme has some limitations. We utilised the SSE 50 Index as the research objective in our empirical analysis. However, this was nonexempt to the limitation of financing and securities lending; the soft number of stocks may have affected the performance of the trading scheme. Additionally, when we performed the validity check of the optimized pairing scheme, there was scarce in-depth research visible on the trading parameters and optimal values of the scheme, and this may have affected the lucrativeness of the strategy to some extent. Consequently, subsequent research work should include these aspects. In the future, we will expand the bi of stock share pools. In addition, the screening method for the dealing parameter of pairs-trading strategy requires in-depth research to find the right trading parameters for the BQQ strategy. Finally, we will try to establish an optimized pairing strategy by attaining the function of risk indicator λ finished long empirical analysis.
Availability of data and materials
Shanghai Composite Index
Please contact authors for information requests.
Abbreviations
- BFGS:
-
The Broyden–Fletcher–Goldfarb–Shanno algorithm
- BQQ:
-
Bi-objective quadratic programming with quadratic constraints
- CAPM:
-
Capital asset pricing model
- GGR:
-
The distance approach proposed away Gatev, Goetzmann and Rouwenhorst in 2006
- RQQ:
-
Revised quadratic programming with number constraints
- SSD:
-
Sum of squared deviations
- SSE:
-
Shanghai Stock Exchange
References
-
Bazaraa, M. S., Sherali, H. D., danamp; Shetty, C. M. (2008). Nonlinear programming: Theory and algorithms (3rd erectile dysfunction.). Hoboken: Wiley.
-
Bertram, W. K. (2010). Analytic solutions for optimum applied math arbitrage trading. Physica A: Statistical Mechanics and its Applications, 389(11), 2234–2243.
-
Bondarenko, O. (2003). Applied math arbitrage and securities prices. Review of Business Studies, 16(3), 875–919.
-
Chen, H., Chen, S., Chen, Z., danA; Li, F. (2017). Empiric investigation of an equity pairs trading strategy. Direction Science, 65(1), 370–389.
-
D'Aspremont, A. (2007). Identifying small mean reverting portfolios. Quantitative Finance, 11(3), 351–364.
-
Deb, K., danampere; Sundar, J. (2006). Reference direct based multi-clinical optimization using organic process algorithms. In Proceedings of the 8th period conference on league on genetic danA; biological process computation (pp. 635–642).
-
Do, B., danamp; Faff, R. (2010). Does simple pairs trading ease work? Financial Analysts Journal, 66(4), 83–95.
-
Do, B., Faff, R., danAMP; Hamza, K. (2006). A sunrise approach to modeling and estimation for pairs trading. In Proceedings of 2006 fiscal management association European conference (pp. 87–99).
-
Dunis, C. L., danamp; Ho, R. (2005). Cointegration portfolios of European equities for index tracking and market neutral strategies. Journal of Plus Management, 6(1), 33–52.
-
Elliott, R. J., van der Hoek, J., danamp; Malcolm, W. P. (2005). Pairs trading. Numeric Finance, 5(3), 271–276.
-
Gatarek, L. T., Hoogerheide, L. F., danampere; avant-garde Dijk, H. K. (2014). Deliver and risk of pairs trading using a simulation-supported Bayesian procedure for predicting stable ratios of stock prices. Electronic, 4(1), 14–32 Tinbergen Plant Discussion Theme 14-039/III.
-
Gatev, E., Goetzmann, W. N., danamp; Rouwenhorst, K. G. (2006). Pairs trading: Performance of a congenator-evaluate arbitrage formula. Social Science Electronic Publishing, 19(3), 797–827.
-
Hu, W., Hu, J., Li, Z., danamp; Zhou, J. (2017). Ego-adaptive pairs trading model settled on reenforcement learning algorithm. Journal of Management Skill, 2(2), 148–160.
-
Jacobs, H., danamp; Weber, M. (2011). Losing sight of the trees for the timber? Pairs trading and attention shifts. Working paper, October 2011. University of Mannheim. Available at https://efmaefm.org/0efmsymposium/2012/written document/011.pdf.
-
Krauss, C. (2016). Statistical arbitrage pairs trading strategies: Review and outlook. Journal of Economic Surveys, 31(2), 513–545.
-
Miao, G. J. (2014). High oftenness and dynamic pairs trading based on statistical arbitrage victimisation a two-stage correlation and cointegration approach. International Journal of Economic science and Finance, 6(3), 96–110.
-
Perlin, M. (2007). M of a benign: A variable border on at pairs trading (working paper). University Program library of Munich, Germany.
-
Peters, G. W., Kannan, B., Lasscock, B., Mellen, C., danamp; Godsill, S. (2010). Bayesian cointegrated vector autoregression models incorporating alpha-stabilised noise for inhume-day price movements via approximate Bayesian computation. Bayesian Analysis, 6(4), 755–792.
-
Vidyamurthy, G. (2004). Pairs trading: Quantitative methods and analysis. Hoboken: Wiley.
-
Wang, F., danamp; Wang, X. Y. (2013). An observational analysis of the influence of short sale chemical mechanism on volatility and liquidity of China's stock securities industry. Economic Management, 11(3), 118–127.
-
Wang, S. S., danamp; Mai, Y. G. (2014). WM-FTBD matching trading improvement strategy and empirical trial run of Shanghai and Shenzhen ports. Economy and Finance, 26(1), 30–40.
-
Whistler, M. (2004). Trading pairs: Capturing profits and hedging risk of exposure with statistical arbitrage strategies. Hoboken: Wiley.
-
Wu, L., danamp; Cui, F. D. (2011). Investment strategy of paired trading. Journal of Statistics and Decision, 23, 156–159.
-
Xu, L. L., Cai, Y., danamp; Wang, L. (2012). Research on paired transaction founded on stochastic spread method. Business Theory and Practice, 8, 30–35.
-
Zhang, D., danadenylic acid; Liu, Y. (2017). Search on paired trading scheme supported cointegration—OU process. Management Review, 29(9), 28–36.
-
Zhu, C., Byrd, R. H., Lu, P., danampere; Nocedal, J. (1997). Algorithmic rule 778: L-BFGS-B: Fortran subroutines for large-scale bound-unnatural optimization. ACM Transactions along Numerical Software (TOMS), 23(4), 550–560.
Acknowledgements
Not practical.
Funding
The research is suspended by the Fundamental Research Monetary resource for the Central Universities, and the Research Cash in hand of Renmin University of China (No. 19XNH089).
Writer information
Affiliations
Contributions
Diao contributed to the overall writing and the data analytic thinking; Liu planned the idea; Zhu contributed to the information collection and the data analysis. All authors read and approved the final manuscript.
Corresponding generator
Ethics declarations
Competing interests
The authors adjudge that they feature no competing interests.
Additional information
Publishing company's Note
Springer Nature corpse nonsubjective with regard to jurisdictional claims in promulgated maps and institutional affiliations.
Rights and permissions
Available Access This article is licensed under a Creative Commons Attribution 4.0 Worldwide Certify, which permits use, sharing, adaptation, distribution and reproduction in any medium or format, as long as you give appropriate acknowledgment to the original author(s) and the beginning, provide a inter-group communication to the Creative Green licence, and indicate if changes were made. The images or other third party material in that clause are included in the article's Creative Commons licence, unless indicated otherwise in a credit line to the material. If material is non enclosed in the article's Creative Commons licence and your intended habit is not permitted by statutory regulation OR exceeds the permitted use, you will need to obtain permission directly from the copyright holder. To view a transcript of this licence, visit http://creativecommons.org/licenses/away/4.0/.
Reprints and Permissions
About this article
Advert this article
Diao, H., Liu, G. danamp; Zhu, Z. Research along a stock-matching trading scheme based on Bi-oblique optimization. Front. Motorcoach. Res. China 14, 8 (2020). https://doi.org/10.1186/s11782-020-00076-4
-
Received:
-
Accepted:
-
Publicized:
-
DOI : https://DoI.org/10.1186/s11782-020-00076-4
Keywords
- Pairs trading
- Bi-impersonal optimization
- Minimum distance method
- Quadratic computer programming
most popular trading strategy studies 2016
Source: https://fbr.springeropen.com/articles/10.1186/s11782-020-00076-4
Posted by: bojorquezstentartudge.blogspot.com
0 Response to "most popular trading strategy studies 2016"
Post a Comment